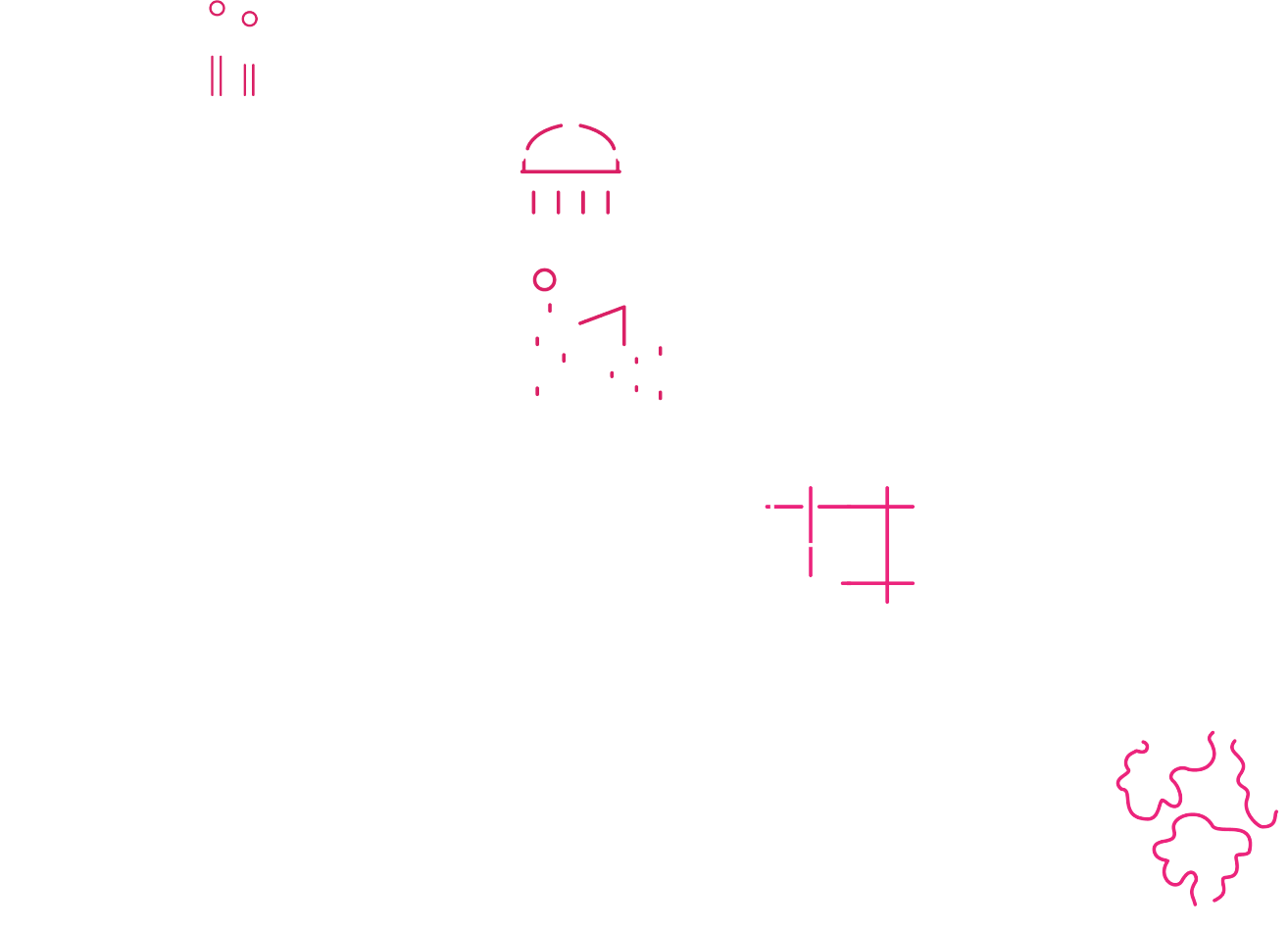
Events
Multimodal machine learning for extreme weather forecasting
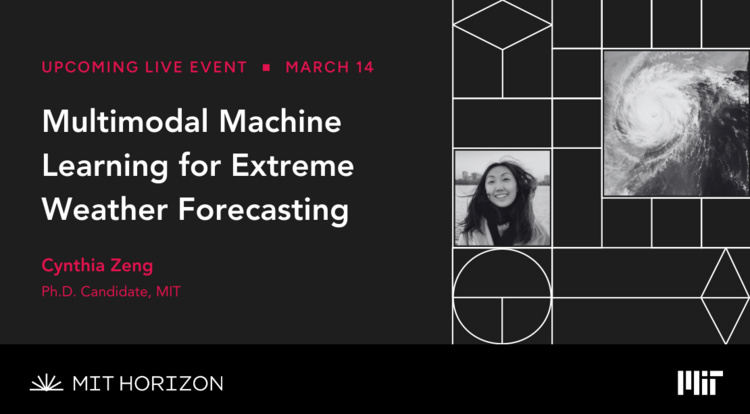
Climate change is escalating the frequency and severity of natural disasters worldwide, necessitating urgent societal adaptation. In this talk, Cynthia Zeng presents a multimodal machine learning framework designed to predict natural disasters. Traditionally, weather forecasting has depended on dynamical equations. However, recent advancements in artificial intelligence are revolutionizing this domain.
The innovative multimodal machine learning framework generates short-term and long-term forecasts by leveraging processing techniques from computer vision, natural language processing, and time series signal processing to integrate various data types, such as satellite imagery, textual information, and tabular data. For 24-hour hurricane forecasting, machine learning models achieve results that are competitive with those produced by national weather forecasting agencies. Artificial intelligence will fundamentally change our interaction with weather, and these machine learning-driven risk assessments will have profound impacts on urban planning, infrastructure investment, renewable energy planning, and insurance policy.